How to Monitor Manufacturing KPIs Automatically with Machine Learning
Last updated on March 24th, 2025
In the high-stakes world of manufacturing, every second counts. When quality issues arise, delays in identifying and addressing the root cause can lead to wasted resources, missed deadlines, and dissatisfied customers. Enter Quality Monitors, a powerful feature in LinePulse that leverages machine learning to monitor KPIs like scrap rate, yield, and throughput in real time.
In this blog, we’ll explore how Quality Monitors work, demonstrate their value through a real-world example, and explain how manufacturing engineers and managers can use them to achieve tangible results.
What are Quality Monitors?
Quality Monitors are a predictive tool designed to track and analyze key performance indicators (KPIs) on the production line. Using historical data and machine learning algorithms, they provide real-time alerts when performance trends in the wrong direction.
Unlike traditional monitoring tools, Quality Monitors pinpoint the specific signals that are statistically correlated, empowering teams to find the root cause quickly and give them a head start on implementing a corrective action.
Here’s a lightning-fast overview of the feature in the LinePulse platform:
How do Quality Monitors work?
The process to set up a Quality Monitor can be broken into three key steps:
- Configuration: Users select a KPI to monitor (e.g., failure rate) and the top 20 signals that affect it.
- Monitoring: LinePulse continuously evaluates the KPI and the signals against historical performance to detect abnormal trends.
- Actionable Insights: When an issue is identified, an alert generates a report which highlights the problematic signals, enabling users to address the root cause quickly.
Quality Monitors in Action
Let’s look at a real example of how a Quality Monitor alert can flag and solve a changing production KPI.
The problem: failure rate increasing
During a production run at an auto manufacturing plant, the yield on one line was at 97%, well within acceptable thresholds. However, a Quality Monitor identified subtle changes in the yield over time and issued an alert. The quality manager received an alert from LinePulse. He opened the Alert Report to see that it had flagged a critical failure rate of 14%—far above the acceptable threshold.
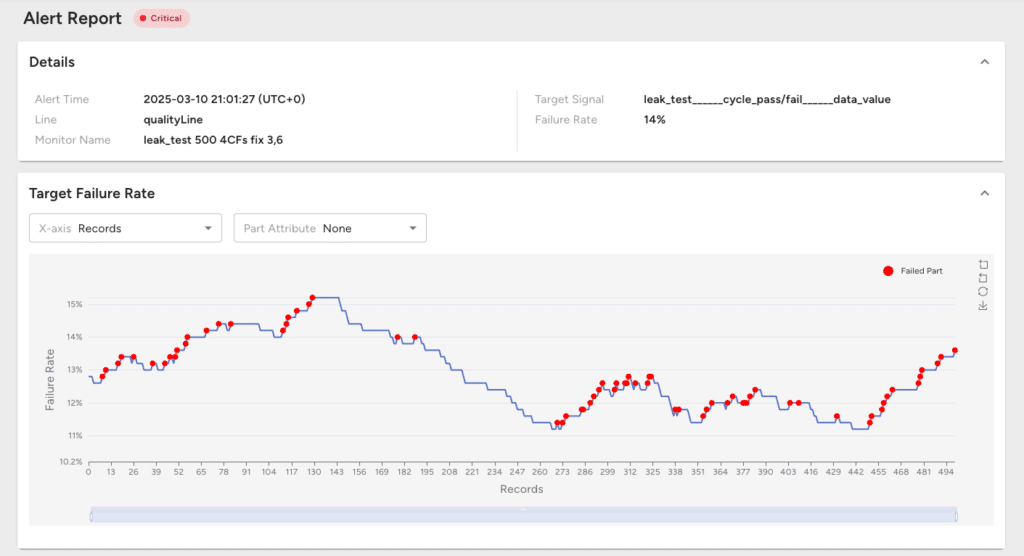
Exploring the results
The quality manager wondered if the failure rate had changed due to a change over in model number. He selected Model Number from the Part Attribute dropdown on the Target Failure Rate Graph to show an overlay with colours that represent the model numbers manufactured during which time.
The overlay revealed that while model changes had been occurring, there wasn’t a clear link between the model numbers and the rate of failure increase. This eliminated variables related to the different models from the possible cause of the high failure rate.
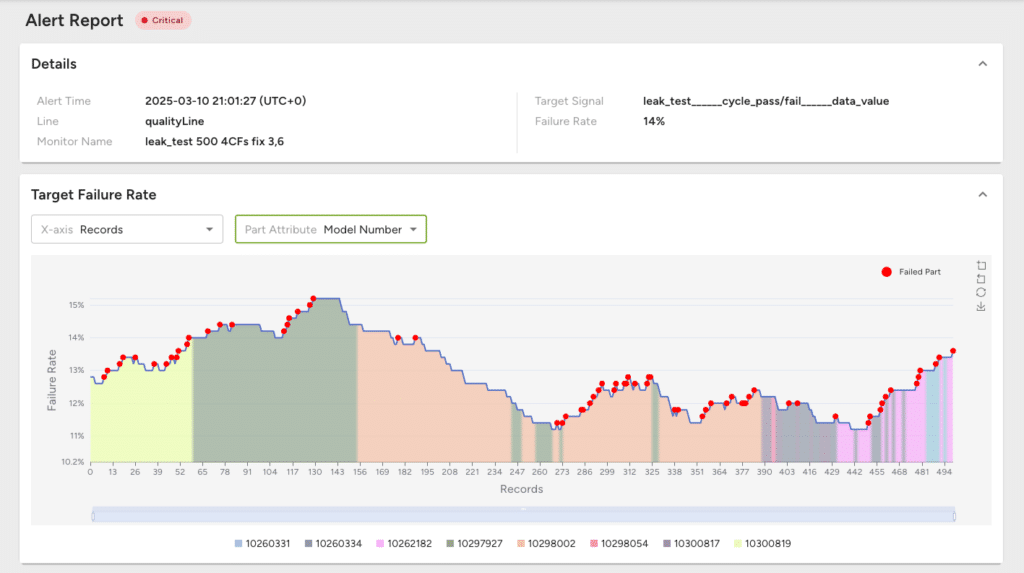
Identifying contributing signals
Scrolling down on the report to the Contributing Features section, the quality manager took a deeper look at the signals that LinePulse identified as being related to the cause of the failure.
By clicking on each of the Contributing Features, he compared the expected signal behavior (green line) with actual behavior (blue line) in the graph. When everything is working as expected, these two lines should overlap. He discovered that the Distance head bearing height signal had deviated significantly from the norm. This was directly linked to the change in yield that triggered the Quality Monitor Alert.
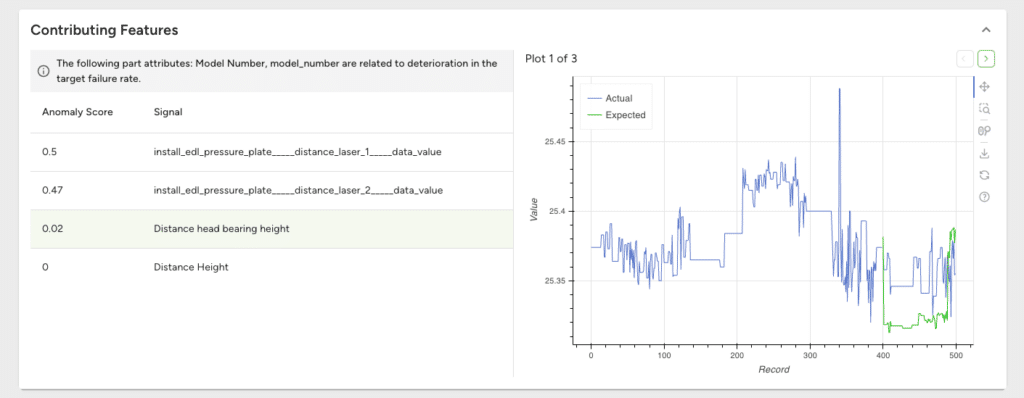
Resolving the issue
Armed with this insight, the quality manager was able to act quickly and alert the team that the Distance head bearing height signal needed attention. After a visual check, the process parameters were adjusted and the yield returned to normal levels.
Quality Monitors for improved KPIs
Quality Monitors are just one module in LinePulse, the problem-solving toolkit for discrete manufacturing. They can be set up at any time to monitor the real time data ingested into LinePulse.
Machine-learning monitoring tools like these are part of the next generation of manufacturing analytics that leverage the vast amounts of data produced by most major manufacturers today to provide insights that help line engineers make fast decisions that improve their production KPIs.